Your session is about to expire
Bias in Research and Why It’s Important to Control for Reliable Clinical Trial Results
What is bias in research?
Research bias definition
In research, bias refers to a systematic error or deviation from the truth, either in the data, results, or conclusions of a study. Bias can originate in any stage of the research process, and is usually related to flaws, incorrect assumptions, or limitations in the study’s design, conduct, analysis, or interpretation/reporting. Different types of bias in research can distort findings and influence the outcomes and validity of a study or lead to inaccurate conclusions. Bias can be entirely unintentional, but can also be intentional, arising from malicious/unethical acts.
All research is subject to bias. Clinical research involving human participants is no exception, but there are a few types of bias that are particularly relevant to clinical trials.
In this article, we will explore the main types of bias in clinical research, why they present a risk to research validity and patient safety, and how to prevent and address bias to ensure scientific integrity in clinical research studies.
Why is bias an issue in research?
Bias has a significant potential to cause issues in research because it compromises the reliability and validity of study findings. When biased data or conclusions are then used in decision-making processes related to healthcare directives and policy, it could lead to the implementation of inappropriate practices or guidelines that can undermine patient care or even pose serious risks to patient health and safety. Thus, minimizing bias in clinical research is important for developing treatments that are safe and effective for patients. It’s the responsibility of clinical researchers to understand the typical sources of bias in research and to take steps to prevent bias, or at the very least aim to limit its impacts and acknowledge any bias when reporting the findings of the study.
What types of research bias affect clinical trials?
The main types of bias in clinical research include selection bias, performance bias, attrition bias, detection bias, and reporting bias. There are other less-common types of research bias that may be relevant to certain clinical research study designs. We describe these types of research bias in the following section, and then provide examples of such types of bias and ideas for preventing it or minimizing its impacts on study outcomes.
10 types of bias in clinical trials and how to address them
1. Selection bias in research
Selection bias is one of the most important types of bias that can skew study outcomes, and occurs when the selection of participants for a study – and/or their assignment to groups – is not sufficiently random. Selection bias can arise from enrolling a sample that is not representative of the broader population, and thus which does not capture sufficient variability in confounding factors. Patients in such a sample may be predisposed to a certain outcome or treatment response due to underlying similarities.
One of the factors that plays into establishing a study’s eligibility criteria is capturing enough variability among participants in order to achieve a somewhat representative sample. This needs to be balanced with limiting extreme variability in confounding variables, in order for the study to have sufficient power to identify a treatment effect. Finding this balance can be tricky, and we’ve discussed it further in the following articles:
Inclusion vs Exclusion Criteria | Power
Clinical Trial Basics: Eligibility Criteria in Clinical Trials | Power
To prevent selection bias, eligibility criteria should be defined in a way that minimizes risk to at-risk populations and eliminates exaggerated variability in confounding factors, while still capturing a sufficient level of diversity in the enrolled sample. Randomization is another important part of minimizing selection bias, as it aims to minimize systematic differences between study arms. Randomization should be carried out strictly according to a randomization protocol that is appropriate for the study, and with proper blinding procedures (allocation concealment) in place.
2. Attrition bias
Attrition bias occurs when there is a high level of patient drop-out, or attrition, wherein the patients who drop out are systematically different from those who remain in the study. The resulting sample differs from the original sample in a systematic way, potentially introducing bias.
Attrition bias can be hard to prevent, as patients may leave a study for numerous and sometimes unpredictable reasons. When a high attrition rate is expected for a study, one potential fix is to over-enroll. Designing patient-centric studies can also make them more attractive and manageable for patients, encouraging engagements and discouraging dropouts.
For more, see Lost to follow-up: Where did the lost patients go? | Power
3. Allocation bias
If the allocation of participants into treatment and control groups is not performed entirely randomly or is influenced by factors other than chance, it can lead to bias in the results. Allocation bias is a form of selection bias, and is prevented by strictly following a randomization protocol that is suitable for the study design at hand, and ensuring that researchers are appropriately blinded during the process, if necessary.
4. Reporting bias
Reporting bias has to do with selective reporting of outcomes or findings from a study. This type of bias is especially prone to having a malicious origin, i.e., dishonest or unethical conduct by researchers in order to reach a certain conclusion about the safety or efficacy of an intervention. However, it can also result from errors in data analysis or accidentally overlooking certain data or possible influencing factors. Reporting bias leads to incomplete or biased dissemination of results, which can have consequences that ripple out through scientific publications and policy-making decisions, potentially impacting patient safety.
Reporting bias is prevented by ensuring ethical conduct and research practices, and by validating data and being careful and attentive to detail during data analysis. Transparency is important for scientific integrity, and that means publishing findings even when they are not what was expected.
5. Performance bias
Performance bias arises when there are differences between the care provided to participants in different study arms which then influences the outcomes being measured.
Utilizing proper allocation concealment, or blinding, for both study participants and researchers (i.e., a double-blind study) can help to limit the potential for differential care decisions or certain patient behaviors based on knowledge about study group assignment.
6. Detection bias
When there are systematic differences in how outcomes are assessed or detected between groups, it can introduce bias into the study findings. One way to avoid detection bias is to blind the analysts who assess the outcomes of the study, as in a triple-blinded study. This works to ensure that any differences are actually due to differences in the intervention received, and not due to knowledge about the assignments affecting the way that the results are interpreted.
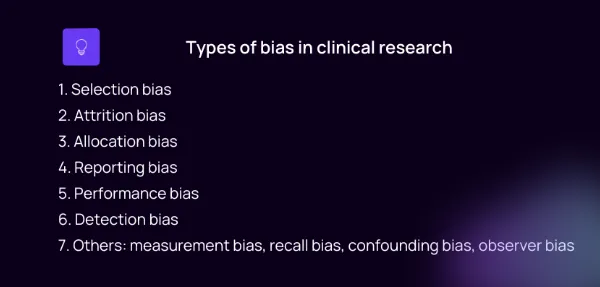
Types of bias in clinical trials continued
The above are the most common sources of bias in clinical research. The list continues below with other types of research bias that may be relevant in certain studies, but they are generally less common/widespread. It’s also important to note that the descriptions may overlap to some degree. Selection bias could be related to attrition or to allocation, for example, and allocation bias could be referred to as a type of selection bias in a given study, depending on nuances in how it’s characterized. What’s important is to be able to recognize the different potential sources of bias and work to mitigate their impacts on study results.
7. Measurement bias
Measurement bias, sometimes called classification bias, describes errors or inaccuracies in the way data is collected, measured, or recorded during a study, which can distort the relationships between variables used to determine study outcomes. Measurement bias can be prevented by developing robust SOPs for data management, making sure they are complied with faithfully, and ensuring proper validation of source data before database lock.
8. Recall bias
Participants' ability to recall past events accurately can fade over time, and can also be influenced by factors such as personal beliefs, emotional states, or other circumstances affecting their behavior during data collection interviews. Recall bias is particularly relevant to retrospective studies and in those employing subjective self-reporting measures that are not collected in the present.
Solutions such as electronic patient-reported outcomes (ePRO) can help prevent recall bias by allowing patients to report remotely on their present state, without having to recall past events or health parameters in scheduled study visits.
9. Confounding bias
Confounding bias refers to the situation wherein a certain correlation between a variable and an outcome is mistaken for a causative relationship, or when a relationship between variables is confounded - blurred - by another variable that was not taken into consideration. In other words, a confounding factor is playing a role in the observed outcome. It is important to identify potential confounding variables (such as patient characteristics and habits, i.e., the influence of smoking in a lung cancer study cannot be overlooked), control for them to the extent possible, and specifically check for confounding relationships during the analysis of study data in order to prevent confounding bias.
10. Observer bias
Observer bias occurs when investigators’ or researchers' expectations, assumptions, or knowledge about group assignments influence their interpretation of study outcomes. Blinding procedures are employed to prevent observer bias arising from investigators or study physicians making differential care decisions for participants in different groups, or from interpreting results differently for the different study arms.
Clinical research bias examples
Let’s take a look at three hypothetical case studies to demonstrate examples of bias in clinical research.
Case 1
An exciting new drug has been developed for depression, and researchers want to know how effective it is. The study sponsor receives approval and begins enrolling patients based on the eligibility criteria. Upon completion, data is analyzed and there is a 50% higher rate of success in the treatment arm compared to the control arm, who took the comparator standard of care drug. However, it’s later found that almost all individuals in the control arm were smokers, while there were few smokers in the treatment arm. A selection bias was thus introduced unintentionally, as researchers overlooked this confounding variable. It’s possible that smoking directly impeded the control drug from exerting a beneficial effect, but it is not possible to retrospectively unravel the influence of this factor, which also represents a potential confounding bias.
Case 2
A clinician at a trial site has not been blinded to participant allocations. When participant A comes in for a study visit complaining of headaches, the clinician decides to prescribe an NSAID (non-steroidal antiinflammatory drug) outside of the study protocol, knowing that participant A has been assigned to the placebo group, and that the supplemental NSAID will help alleviate the headaches and will not interact with the study drug. Participant A then responds to a study questionnaire, revealing that they did not have headaches all week. This act of differential care by the physician represents performance bias, and may skew study results by hiding a side effect experienced by participant A in the placebo group.
Case 3
A new study is enrolling participants for a promising new cancer drug. During the informed consent procedure, the principal investigator is touched by a patient’s difficult story, and decides that the individual deserves to be in the treatment group. The PI then modifies the randomization procedure to ensure this patient is assigned to the treatment group and thus receives treatment. This exemplifies allocation bias, a type of selection bias wherein the assumption of completely random allocation is broken. While it is not guaranteed to skew study results, it has the potential to introduce bias, and this action goes against ethical and impartial practices in research.
How to avoid bias in research
Preventing bias in clinical research largely begins with a well thought-out study protocol that is designed from the bottom up to prevent the introduction of bias. However, careful adherence to the study protocol, SOPs, and regulations and ethical guidelines throughout the trial is also important for limiting further sources of bias.
- Thoughtful study design
Time should be taken to develop robust, patient-centric study protocols that have clear objectives, cleverly selected inclusion criteria, an appropriate randomization procedure, blinding protocols, and standardized data collection methods and data handling protocols. Most bias is prevented in the initial design stage of the trial.
- Sample size calculation
A sufficient number of participants should be included in the study to achieve enough statistical power to answer the research hypothesis and maximize the generalizability of findings. This should be balanced with a consideration of the extra resources required per additional patient enrolled, along with the ethical consideration of exposing participants to any risk inherent to the study.
- Inclusion and exclusion criteria
The inclusion criteria and exclusion criteria should be set in a way that supports enough variability in the study population to mimic that of the broader population, while avoiding overly extreme variability in confounding characteristics that could make it impossible to reveal a drug effect.
- Blinding procedures
If there is any reasonable potential for bias to be introduced in the case that either researchers or patients should become aware of group allocations, blinding measures should be implemented. Most studies are either single-blind (participants blinded) or double-blind (participants and researchers blinded), but triple-blinding (also blinding the analysts) can also be used when there is potential for bias during analysis of study data. Unblinded studies, also called open-label trials, are less common in controlled trials, instead being mostly reserved for single-arm trials, wherein there is no grouping at all and thus blinding is unnecessary.
- Randomization techniques
Employing a randomization method that is appropriate for the study helps to distribute potential confounding variables evenly among study groups. We’ve written about various types of randomization protocols here.
- Transparency in reporting
As part of ethical conduct in clinical trials, researchers and sponsors should prioritize being transparent in their reporting of study methodologies, data analysis, and interpretation of results, along with limitations of the study and details about any potential conflicts of interest. Transparency is important for upholding scientific integrity and public trust in clinical research, but also helps to reveal potential biases that may have been missed. The idea behind transparency in research is to accept that mistakes are natural, and that it’s better to reveal and acknowledge them in order to rectify research findings and ensure that misleading results are not used to inform healthcare guidelines or policy, which could pose a threat to public health.
- Independent review
Clinical research protocols are reviewed by an IRB to ensure they meet ethical guidelines. Part of the independent review process also focuses on identifying any methodological flaws or potential sources of biases that could skew results or lead to inaccurate findings.
Conclusion
There are many potential sources of bias in research, and bias in clinical research carries potential risks to the safety and well-being of patients and the general public. Minimizing clinical research bias is a major priority in study design and conduct, and it’s important for trial sponsors and clinical researchers to understand potential sources of bias in order to recognize and prevent them. When bias cannot be avoided, it should at the very least be mitigated to the extent possible and acknowledged during the reporting of findings, which will support the generation of reliable evidence to support transparently informed decision-making for patient care and healthcare policies.