Your session is about to expire
Overview: The Current Landscape of Machine Learning Clinical Trials
What is machine learning (ML) in clinical research?
Machine learning (ML) is a subset of artificial intelligence (AI), which uses algorithms and statistical models to automate analysis and allow “self-learning,” i.e., improvements in the model even without human input.
ML can help researchers recognize patterns in large collected data sets, and has the potential to enhance trial quality and efficiency in various ways. ML has also been utilized directly in medical interventions which have been studied in randomized controlled clinical trials.[1] There are thus many different ways in which ML is being incorporated into clinical research, but as we will discuss in this article, the technology is still relatively early in its adoption and there are barriers and challenges to be resolved if it is to be used ethically and bring about tangible benefits for patients and researchers.
How is machine learning used in clinical trials?
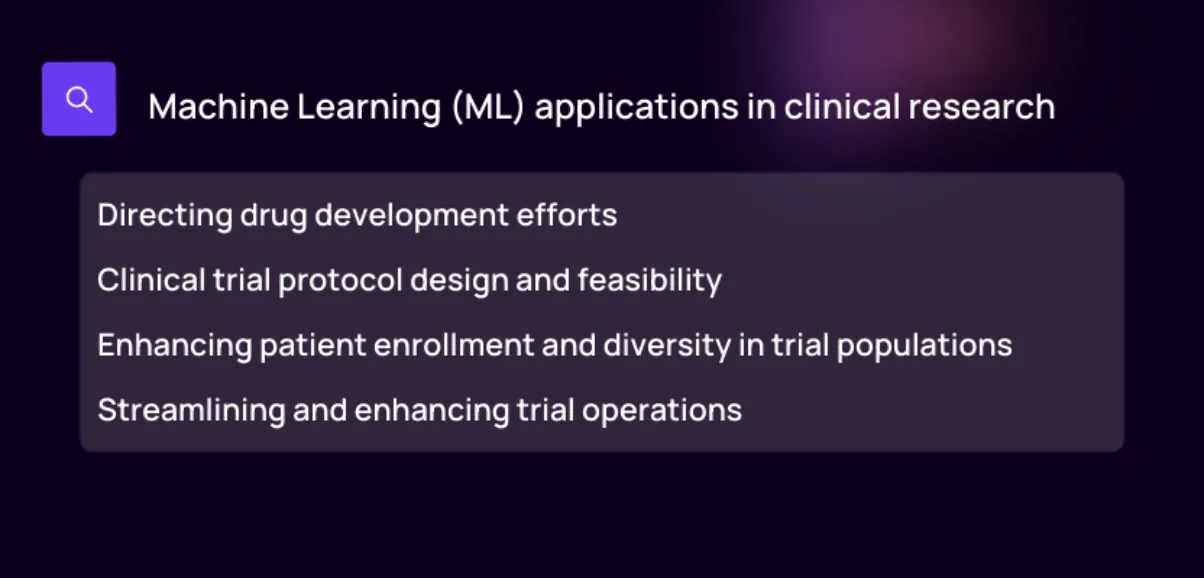
In the context of clinical research, ML has already been utilized to assist with an exciting variety of tasks and operations in both drug development and clinical trial operations. Some of the current applications of machine learning in clinical research are detailed below.
Directing drug development efforts
In the context of drug development efforts, machine learning algorithms have been trained to help identify and suggest candidate molecules for specific targets or conditions through high-throughput screening.[2] Drug candidates can be further explored and rationalized through predictive toxicology.[3]
To guide the design of candidate drug molecules, ML algorithms can also explore and identify novel targets and biomarkers by efficiently filtering through enormous sets of genomic data and providing high-resolution insights into the data.[4]
Clinical trial protocol design and feasibility
Machine learning has been applied to streamlining clinical trial protocol design, for example with automated adaptive algorithms which would be laborious to explore manually, and to facilitating feasibility studies by predicting the likelihood of early termination of clinical trials.[5]
Enhancing patient enrollment and diversity in trial populations
A prominent utility of machine learning lies in identifying eligible candidates from large patient databases, particularly for remote/decentralized clinical trials and international studies, where the potential patient populations are much more diverse and widespread - and for whom health records may be in various disparate formats that are significantly harder to aggregate and filter through manually. Prospective populations can be further refined through ML as per eligibility criteria and protocol specifics, for example by using risk stratification to determine who is fit for the trial interventions.
This application also has potential implications in improving diversity in trial populations, as the model can be trained to maximize diversity or include (and even first identify) certain under-represented groups.[4] This is currently a prominent topic as diversity is exceedingly important in order for the results of controlled trials and eventually approved therapies to be appropriate for the broader population, yet there has classically been disproportionate inclusion of certain groups in clinical research.[6]
Streamlining and enhancing trial operations
In terms of the actual conduct of clinical trials, machine learning is positioned to enhance and optimize various workflows from study start-up through to data analysis and reporting. ML has been used to enhance site selection by analyzing RWD and RWE from sites and local patient databases, helping sponsors accurately identify sites with relevant patient populations and high enrollment potential.[4] It has been applied to evaluating the safety of new treatments with higher precision through deeper analysis of adverse events data, and to assessing the efficacy of the study drug by enhanced analysis of health endpoints, medical images, etc.
Machine learning has also been applied to real-time patient monitoring and tracking of trial progress with advanced analytics that help sponsors detect potential problems before they actually occur.
This list is not exhaustive, yet it’s already clear that machine learning has significant potential for improving clinical trial operations and trial quality. Nonetheless, there are still limitations to the implementation of ML in each of the aforementioned aspects, along with ethical considerations that should be taken into account. In the next section we will discuss some of the current challenges with machine learning in clinical trial applications.
Current issues related to machine learning clinical trials
Setbacks in applications of machine learning algorithms in the clinical context have commonly been attributable to factors such as the following:[1]
- Lack of generalizability due to training on specific subsets of patient data
- Inflexibility in terms of adapting to a new context when the system is trained with data from a singular context
- Inability to demonstrate a clinically meaningful benefit
The use of machine learning in clinical trials also carries some degree of risk related to privacy protection - sensitive personal data may be leaked or exposed if proper security measures are not implemented within the system.[3] This also requires thorough training of all staff interacting with the system.
Further, although bias is not unique to clinical trials employing ML, there is the risk of the introduction or perpetuation of bias through the use of ML.[3] Care must be taken to train the model on datasets that are relevant and appropriate to the study population, and not to generalize the findings to populations that may not be properly considered in the original model without duly addressing this potential limitation. An ideal way to combat bias, whether using ML or not, is to focus on generating high-quality data in sufficient quantity, which begins with the design of the study protocol and sample size selection.[3] On the flip side, bias reduction is actually another area of implementation of ML.[3] As with most technologies, the biggest question relates to how and for what purpose it’s implemented.
Future directions of machine learning in clinical trials
As AI and ML technologies continue to advance, so will their application towards improving clinical research. Emerging uses of machine learning in clinical trials include integrated analysis (incorporating numerous interconnected data sources), personalized therapy or treatments assignments aided by precision medicine, adaptive trial designs, and the use of natural language processing to open up new possibilities for remote patient-reported outcomes and electronic data capture.[7],[8]
Other advancements will likely relate to the transparency of machine learning models, which are currently largely treated as “black boxes.”[7] Transparency in AI and ML algorithms will be an important factor in addressing and rectifying bias in clinical studies. Separate technologies may also be integrated for even more powerful and traceable trial workflows; for example, blockchain could be used to ensure greater trustworthiness by providing non-editable audit trails of data handling steps undertaken by all stakeholders involved in a study, including those related to the training and outputs of ML models.[7]
With machine learning, clinical trials can reach new levels of power and efficiency, but it is important to deliberately address concerns relating to patient privacy and confidentiality, model transparency and security, and bias arising from training datasets or model design.
Conclusion
In summary, machine learning has already proven to be useful in an array of different areas within clinical research, from data collection and analysis to patient recruitment and retention, but there are still developments to be made before it reaches its full potential. While challenges remain regarding privacy protection and bias, ongoing and emerging developments provide hope for new opportunities that could lead us into a future where many of the time-consuming and routine tasks associated with conducting clinical trials are simplified with AI and ML-based solutions.