Your session is about to expire
Accelerating Toward New Paradigms: Next Generation Clinical Research and Evidence Based Medicine
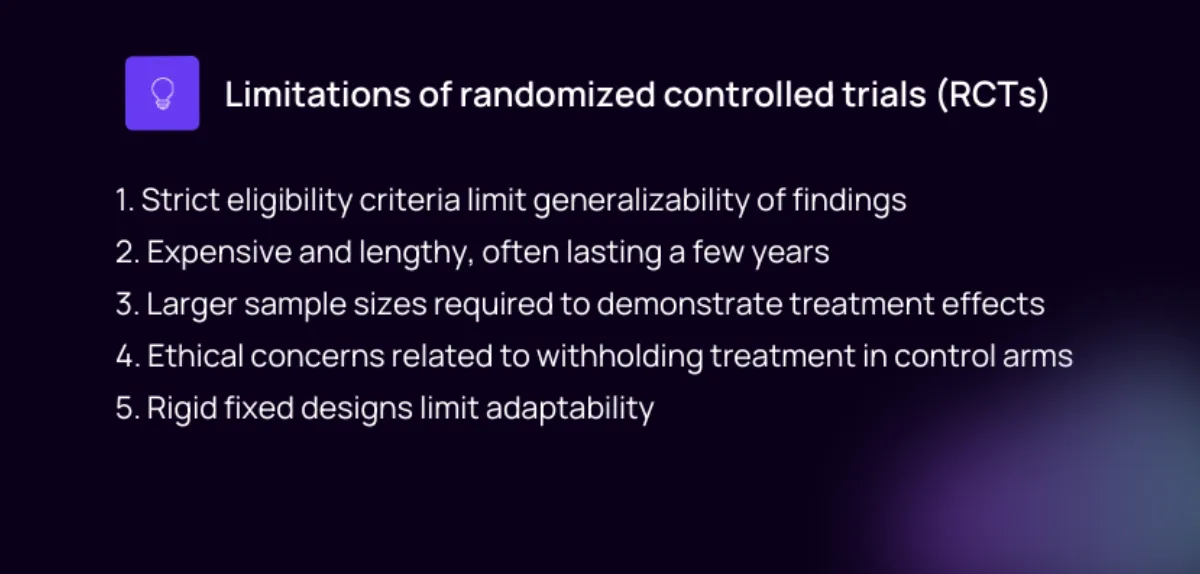
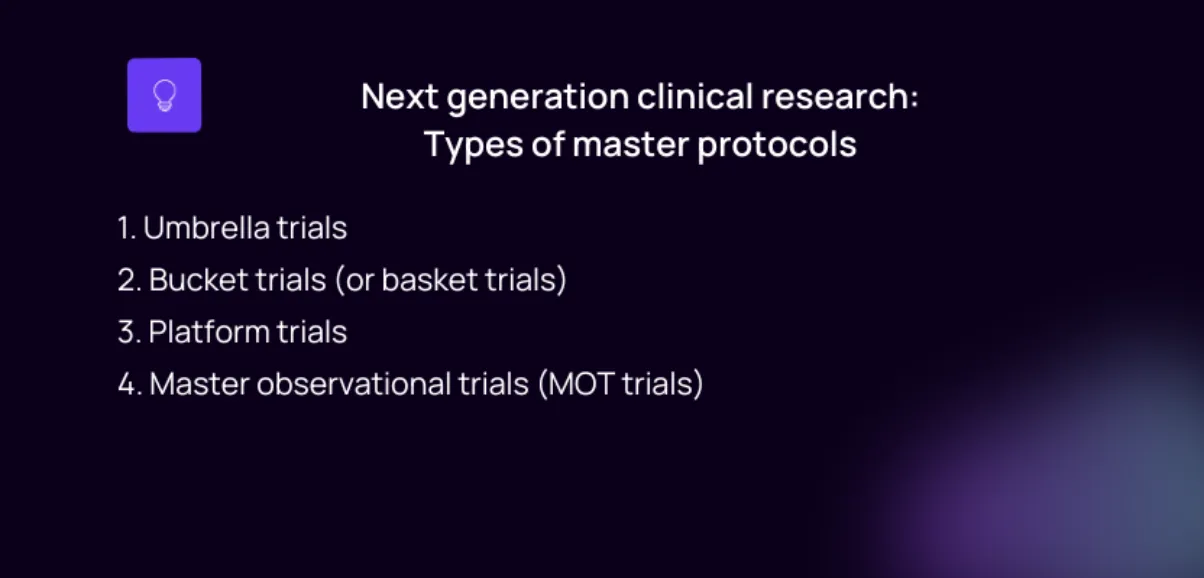
Other Trials to Consider
Popular Categories
Popular Guides
Learn More About These Treatments
At Power, we believe in using data responsibly to help you find the right clinical trials — without compromising your privacy. This page explains how we use cookies and personal data across www.withpower.com.
Before You Create a Profile
When you browse Power's website, you're opting in to our use of cookies. Cookies are used to improve your experience and help us understand how the site is used so that we can make improvements for you in the future. Specifically, we use cookies to:
Personalize Your Experience
We use cookies to customize your visit based on basic information like your general location (determined by your IP address). This allows us to:
- Show you clinical trials that are geographically relevant to you
- Tailor search results to match the conditions or keywords you've explored before
- Pre-fill certain fields or remember your previous searches, so you don't have to repeat them
Save Your Preferences
We remember what you interact with during your visit — for example:
- The conditions you search for
- Whether you prefer certain types of studies (e.g., paid trials, trials for a specific age group)
- Your sorting or filtering preferences when browsing trials
This helps us make your experience more efficient and personalized the next time you visit.
Understand How the Site Is Used
Cookies help us collect anonymous usage data so we can make Power better. We use these insights to:
- Monitor how users move through the site — for example, which pages get the most traffic and where users tend to exit
- Track how long visitors stay on each page and whether they find what they’re looking for
- Identify points of friction or confusion so we can improve usability
- Test design changes (like different page layouts or buttons) and measure which version performs better
- Detect and fix bugs or slow-loading pages to maintain site reliability
These analytics are aggregated and do not include personal identifiers. We use tools like Google Analytics to process this data, but we don't use it to target ads or sell your information.
We do not:
- Sell or share your personal data with advertisers
- Use your behavior on our site to target you with third-party ads
All cookie use is designed to support your experience on Power — never to track you across the internet or monetize your information.
After You Create a Profile
When you sign up for a Power account, you agree to our Privacy Policy and Terms of Service. Creating a profile allows us to better serve you by tailoring the platform to your specific needs.
Once you create a profile:
- We may collect additional information about your health and clinical interests to help us match you to the most relevant studies.
- We continue to use cookies to remember your session, keep you logged in, and personalize your dashboard.
- You have full control — you can delete your profile at any time, and we'll remove your personal data in accordance with our privacy practices.
We use your data solely to fulfill our mission: helping you find clinical trials that could be a fit — not for advertising or resale.