Your session is about to expire
Clinical Trial Data Management
What is clinical trial data management?
In clinical research, data management refers to the processing of trial data - patient information and all other data collected throughout the entire trial - which includes its collection, organization, storage, validation, and analysis. Good data management practices are central to a successful and compliant trial.
A 2011 study published in the Journal of Clinical Epidemiology investigated the effect of electronic data capture (EDC) and demonstrated that advanced clinical trial data management systems could reduce data entry time and cut data cleaning and analysis efforts by half. Further, they were found to reduce the time required for database lock by up to 40%.[1] Since this study was published over a decade ago, it precedes many of the further advancements in data management solutions that have made clinical trials more efficient over the past years.
Today, clinical data management systems, or CDMS, are widely used by sponsors and sites to help them improve patient safety, increase data accuracy, and streamline operations overall.[2] These will likely become increasingly important considering that 97% of companies surveyed in the Tufts eClinical Landscape Study indicated that they planned to incorporate more clinical data from a wider variety of sources over the following years.[3] Nevertheless, 77% of companies surveyed in the same study reported difficulties entering data into their electronic data capture (EDC) systems, revealing that there is still progress to be made.[3] In this article, we will take a deep dive into the world of clinical trial data management.
Why is clinical data management important?
Effective data management practices in clinical research are important for the following reasons:
- Improving data quality: Standardized processes for the collection, organization, validation, and cleaning of data can reduce errors and improve the overall quality of trial data.[4] This improved accuracy can lead to improved patient safety, regulatory compliance, and results.
- Enhancing compliance: Complying with the requirements of regulatory bodies such as the FDA and EMA is an essential yet challenging aspect of running clinical trials. Solid data management systems help with streamlining data processing and improving accuracy through automated edit checks and integration with standards, further acting to provide audit trails and proof of compliance.
- Ensuring patient safety and privacy: Proper data handling is essential for maintaining privacy and confidentiality of sensitive personal data of trial participants. Further, accurate data management ensures patient safety by allowing efficient monitoring and timely identification of adverse events.
- Timeline management: Efficient data management tools can reduce the time and efforts required for data processing. This can translate into lower manual workload for researchers when implemented well, and subsequently shortened time to database lock and faster time to market for the investigational product.
What are the roles and responsibilities in clinical trial data management?
There are many individuals across different teams involved in diverse trial operations who may create, access, transfer, or use trial data in some form or another throughout a study.[5] Assigning roles and permissions for data access is an important aspect of good data management practices. Each of these individuals should be properly trained on data privacy and data handling procedures, for example through SOPs for data processing.
Typically, a trial will involve a data management team consisting of a data manager along with other roles which are outlined below (note that this is a generalization; each trial/sponsor organization may have its own specific internal structure):[2]
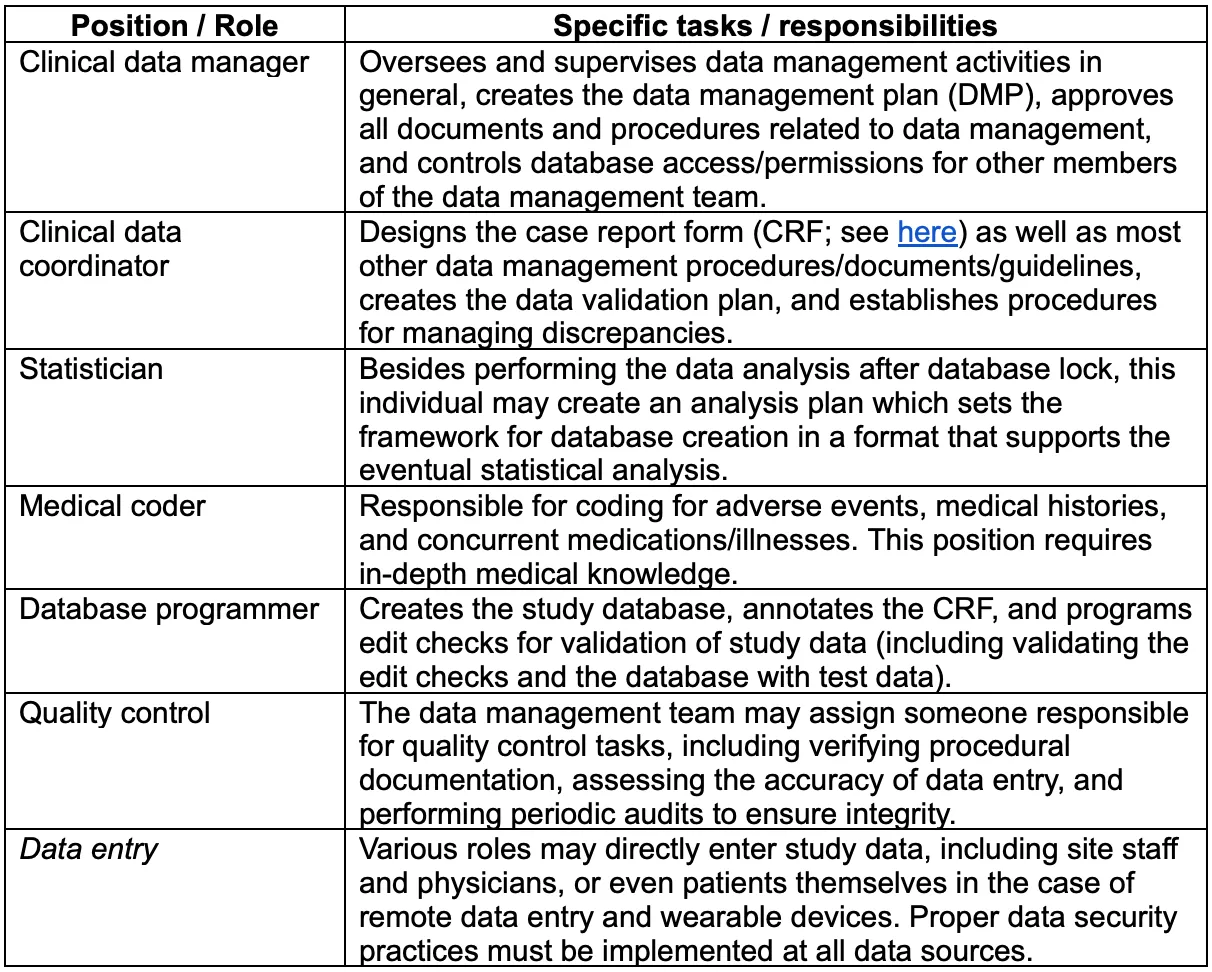
The data manager is responsible for creating and overseeing implementation of the data management plan, or DMP, the set of guidelines that specify procedures to be followed as well as the use of any clinical data management system (CDMS) or other technological tools. The data manager is also ultimately responsible for ethical and regulatory aspects of trail data processing.[6],[7]
How is clinical data handled in clinical trials?
Clinical data is typically handled according to guidelines set forth in the data management plan (DMP), a document or set of documents outlining data handling and processing procedures to be followed throughout a clinical trial. The DMP may consist of multiple standard operating procedures (SOPs), which will depend on the institution/sponsor's internal structures and the nature of the trial and its data. All staff and personnel interacting with sensitive data should be thoroughly trained on these SOPs.
Clinical data management plans cover all crucial steps of a clinical research project, incorporating the overall study timeline as well as interim goals to be accomplished by different teams. It is also important to identify and incorporate data regulations standards into the DMP. A template can be helpful for keeping track of all such requirements, and facilitate faster start-up across studies that vary in the details but which can use the same general DMP template.[7] Although regulatory agencies do not explicitly mandate the use of a DMP, they often expect it and look to audit it, so it is considered best practice to create and implement a comprehensive DMP.[7]
What should a data management plan (DMP) include?
The DMP should include considerations of all aspects of data collection (including designing the case report forms and the database), validation and auditing, storage, and analysis. It should be exhaustive in that it covers data handling across all trial operations and throughout the entire timeline of the trial.
The DMP acts as a master guideline for everyone involved in the trial’s operations, but also as an overview of the trial for stakeholders. Importantly, the DMP should be treated as a living document - it can and may be need to be updated and changed at one or more points throughout the trial, depending on roadblocks encountered or possible changes to protocol.[8]
The DMP usually includes specifications on:[9]
- Roles and responsibilities: Outlines the key actors involved in the DMP and their specific roles.
- Data types: Types of data to be analyzed, which could include patient baseline characteristics, health endpoint measures, time-to-event, etc.
- Data format: The format of data and any metadata, including units and field constraints to ensure data is captured in the proper format.
- Access, sharing, and privacy: Permissions and access for different users of the data management system and interfaces must be defined and strictly managed.
- Redistribution policies: Clear definitions on usage rights of the trial data, both for the current trial and for future research.
- Storage and security: Instructions for how the data is to be stored in a way that maximizes security while still optimizing accessibility.
- Budget: Budget allocation to data management tasks and operations, which may vary by trial stage.
What are the steps involved in data management?
Clinical data management is an involved process requiring significant organization and attention to detail. The initial steps of CDM begin before enrollment starts, and involve careful planning.
1. Plan and design
The first step of clinical data management requires thorough planning according to the trial protocol and the specific data types involved. Before the trial begins (i.e., before recruitment), the following should be established:[2]
- Databases: The database should take into account the specific types of data that will be involved in the study, and should be designed to accommodate this data in a clear and accurate way. Edit checks should be programmed in to automatically catch errors, missing data, or redundancies, thus keeping the data clean during the trial and avoiding the need for a lengthy cleaning process at the end of data collection.
- Case report forms (CRFs): The case report form, or CRF, serves as the template for the collection of patient data. Fields should be constrained such that data is collected coherently between different devices/interfaces and study sites/personnel. The design of the CRF is specific to the trial at hand, and the CRF should be annotated in order to facilitate data processing and analysis. The CRF can be populated from various data sources, including EDC devices, ePRO, and wearables - all interfaces used for data collection must be validated, for accuracy and functionality as well as user-friendliness, before the study begins.
- Data management plan (DMP): The data management plan should be thorough and requires consideration of many aspects of trial operations as per the trial’s unique protocol. The DMP will likely involve the creation or updating of SOPs for specific tasks, and should include explicit protocols for discrepancy resolution. Individual roles and tasks must be explicitly and clearly assigned to the data management team and anyone else interacting with the study data, and this may involve training to ensure everyone is up to date on the latest practices and regulations. Any clinical data management system (CDMS) or other software tools must be set up and validated at this time, and user permissions need to be set to ensure data privacy and compliance with regulations such as the HIPAA Privacy Rule and Security Rule.
2. Quality assurance and user acceptance testing (UAT)
The data management system, including protocols and guideline documents, should be validated before launch but also periodically checked for continued validity, compliance, and functionality. For this last point, end users of the system should provide input on the usability of the system and demonstrate familiarity with its operation. End users can be members of the data management team, but also site staff and even participants - again, it depends on the design of the trial and the data collection methods and endpoints used.
Data entry fields should be complete, constrained where needed to ensure consistency, and properly coded to facilitate analysis. CRFs can be populated with dummy data, and edit checks can be tested by entering known errors and making sure they are caught. Even protocols and guidelines can be validated through simulations. Once all aspects of the database and the data management system are established and validated, the data collection process can begin.
3. Data collection
Data collection can be performed via various methods, again depending on the specifics of the trial protocol. Data can be entered onto paper CRFs by site staff during study visits and then transcribed into the CDMS or EDC, or data could be inputted directly into the CDMS via EDC or eCRFs (electronic case report forms), either by the site staff or by the patient themselves in the case of decentralized trials. In comparison to traditional paper-based data collection, electronic data collection allows for a quicker collection of data and increased accuracy, saving researchers time and manual data entry tasks - although this is dependent on good implementation of the electronic systems and proper training of staff.
4. CRF monitoring
CRFs may be monitored throughout the trial, usually by a clinical research associate (CRA), and completed forms are sent to the data manager. These forms are tracked by the data management team in order to take note of issues and resolve them in a timely manner.
5. Medical coding and SAE reconciliation
Medical coding works to standardize data collection and facilitate analysis by ensuring consistency. Medical terminology, adverse events, co-illnesses, discrepancies, deviations, etc. are labeled with consistent notation as defined during the medical coding step. Standardization is key to data analysis.
To illustrate medical coding in simple terms, let’s imagine that one site reports an adverse event in a patient presenting with kidney stones. Another site may report the same adverse event using the medical term nephrolithiasis. Standardizing the reporting of kidney stones under a coherent notation scheme (let’s say AE_K, as a hypothetical example) will ensure that this adverse event is noticed quickly as well as facilitate pattern identification and data analysis. In this hypothetical example, AE indicates that it is an adverse event suspected to be related to the investigational product, causing it to be registered accordingly in the system (under adverse events).
6. Data validation
Any data entered into the CDMS or other electronic data entry systems will usually be subjected to edit checks (programs written to automatically validate data against field constraints defined in the DMP). Nevertheless, there may be additional data validation measures set in place, such as periodic audits or manual revisions to ensure the absence of other errors, inconsistencies, or missing data.
7. Discrepancy management
Any discrepancies identified in the data or the system will be dealt with as per guidelines set forth in the DMP and relevant SOPs. Depending on the source of the discrepancy or error, it may necessitate further investigation, such as on-site monitoring or source data verification (SDV). In some cases, data discrepancies may be solved directly by the data manager.
8. Data de-identification
An important step for patient privacy and confidentiality (and thus regulatory compliance in these regards) is de-identifying study data before analysis. Data or attributes that are considered PHI or PII should be removed or coded to protect patient confidentiality.
9. Database lock
Once data collection is finalized and the data has been thoroughly validated, de-identified, and cleaned, the database is “locked” from further editing. The database can only be locked when final approvals have been given by all relevant stakeholders. When well-implemented, electronic data management systems can come in handy for minimizing the time to database lock.
10. Statistical analysis
Finally, the locked data set is analyzed by statistician(s) to identify trends and hopefully uncover clear indications of the safety and/or efficacy of the investigational product. The analysis process depends on the study design (i.e., randomization, treatment allocation, etc.) as well as the hypothesis - the goal is typically to either prove or disprove one or more of the research hypotheses according to a predetermined level of significance.
Compliance and data privacy and security in clinical trials
Patient privacy and confidentiality are vital underlying themes in clinical research, which are closely tied-in to regulatory requirements. Researchers must keep private patient information securely stored and use it only for authorized purposes. The process begins with informed consent, wherein the patient should be informed explicitly about what personal data will be collected and how it will be stored and used for the trial, in order for them to make an informed decision to participate and accept the stated uses of their personal data. Some patients risk consequences in their personal lives in the case of data breaches, and non-compliance also carries penalties/fines for the sponsor and/or site, so this aspect of data management must be taken seriously.
Without going into too much detail (you can find out more about specific regulations here), the principal regulations that govern the use of patient data in clinical research are ICH E6 (R2), i.e., Good Clinical Practice (GCP; in the US and the EU), the HIPAA Privacy Rule and the HIPAA Security Rule (US), the HITECH act (US), and the GDPR and CTR (in the EU). These regulations impose different types of standards for the processing of “sensitive” information - that which can be used to identify a patient or reveal things about their health condition - which are captured under the definitions of personally identifiable information (PII) and a subset of PII known as personal health information (PHI).
Key considerations for appropriately addressing patient privacy and data confidentiality and security in the data management plan include:
- Clearly define roles and responsibilities, including permissions and access to health data and different areas of the data management systems.
- Educate and inform all team members on the importance of privacy and best practices for complying with data privacy regulations. Maintain training records to help keep track of competencies and serve as proof of compliance.
- Conduct regular risk assessments of data management systems to catch any potential gaps in security and avoid breaches.
- Strictly implement security measures to restrict unauthorized access to trial data.
- Ensure compliance with GCP, HIPAA, and any other applicable regulations.
- Perform regular monitoring activities.
It is good practice to directly incorporate aspects of the different regulations directly into any data management system used in a trial, as this can help automate error identification and resolution and will save manual revision down the line. This will further assist with demonstrating compliance with regulatory bodies.
What is SDTM in clinical data management?
SDTM, or the study data tabulation model, is a standardized data table format used for regulatory submissions with the FDA in the US. This standard was introduced by the Clinical Data Interchange Standards Consortium (CDISC) to streamline and facilitate the collection, analysis, management, exchange, and reporting of clinical study data.
It is important to consider SDTM when creating the DMP and the study database. When using a third-party CDMS software, look for a system that supports SDTM integration.
Implementation guidelines: Clinical data management process flow chart
Below is a graphical representation of the steps involved in clinical trial data management operations.
Leveraging technology to streamline clinical data management: CDMS, eCRF, EDC
An increasing proportion of trial sponsors and sites are moving away from paper-based data collection and entry systems and towards electronic data management tools. This necessitates flexibility and additional training for the adoption of new methods and software into workflows (and the transition may take some time), but these electronic tools offer several advantages when properly implemented, as they:
- Improve efficiency and increase productivity by reducing the time spent collecting, entering, cleaning, and processing data.
- Ensure high quality and accuracy of clinical trial data by significantly reducing errors and inconsistencies, often through automated validation.
- Streamline regulatory integrations, which promotes the security and privacy of data and facilitates demonstration of compliance.
- Enable real-time monitoring, which is powerful for quick identification and resolution of issues, as well as ensuring patient safety by noting adverse events in real-time.
- Facilitate sharing and data transfer, improving collaboration between teams and between sites and sponsors, while maintaining data security.
Three of the principal technological solutions utilized in electronic data management in clinical research are:
- CDMS: Clinical data management systems are software applications designed to support clinical research by facilitating all aspects of data management, from database design through to analysis. CDMS often integrate directly with other tools as well as regulatory standards.
- eCRFs: Electronic case report forms are simply electronic versions of case report forms, which offer advantages in terms of data accuracy (constraints, edit checks, coding assistance, etc.) as well as smooth integration with CDMS and data collection tools (see next).
- EDC: Electronic data capture is a broad term referring to the direct collection of source data in electronic format, i.e., rather than collecting data on paper and then transcribing it into the CDMS. EDC may utilize or integrate with tools such as electronic patient reported outcomes (ePRO, such as remote patient surveys) and wearable devices, and are typically connected to eCRFs so that data is simultaneously registered in the case report forms as well as the CDMS.
There is a vast offer of clinical data management software solutions and tools available on the market, both ready-made and customizable, and offering intuitive integration with other systems that may already be in your workflows (be sure to check this last point explicitly, as the reality of cross-platform integration appears to be somewhat behind the claims, currently).
Conclusion
Efficient clinical data management practices are essential for the quality and accuracy of trial results, regulatory compliance, and for patient privacy and safety. Overall, solid data management can shave days or weeks off trial timelines, helping bring new drugs and treatments to patients faster.