Your session is about to expire
What is Source Data Verification (SDV)?
In clinical trials, source data verification—SDV—is the process of validating source data.
What is source data in a clinical trial?
Source data is defined by the ICH as “all information in original records and certified copies of original records of clinical findings, observations, or other activities in a clinical trial necessary for the reconstruction and evaluation of the trial.”
Source data is contained in source documents, which are either the original records (from the first time data is recorded/created) or certified copies of those records. Source data refers to the original data upon which the trial and its assessments are based. Source data can include:
- Study protocols and other trial documentation
- Communications and promotional material
- Patient-reported data (from study visits, remote data collection or EDC tools, surveys/questionnaires, etc.)
- Laboratory results, medical images, and health data collected by the study clinician or a trial nurse
- Electronic health records
As the name implies, source data verification (SDV) is the process of verifying source data for accuracy.
Why is source data verification (SDV) important?
Source data verification serves as an important quality control step to ensure the integrity and accuracy of study data, and also forms part of the regulatory requirements for clinical trials.
SDV serves to confirm that data has been entered accurately into electronic records such as eCRFs or a CTMS, catching and correcting errors in the study data before analysis. It also helps ensure physical records are well-organized and accessible, which facilitates the recall and use of the data internally and the auditing of this data by external auditors. SDV can involve, for example, comparing a paper chart from a patient's first study visit to the electronic CRF, or checking data fields from CRFs against the study database before data lock. If there are discrepancies found between documents, or if necessary records are missing, such issues would be resolved during SDV.
It is generally accepted that SDV can improve the quality of clinical trials by ensuring the integrity of the data, and therefore that of the results as well. Although it can be time-consuming, SDV can reduce the costs associated with serious errors in the data and the resultant delays or compliance issues.
For this reason, SDV is generally done as part of a quality control process known as data cleaning, which occurs before the study database is locked for analysis. Data cleaning involves scrutinizing data sets to identify missing data, outliers, or other errors that may have been made during manual data transcription or due to corrupt file systems, errors in software code, etc.
What does SDV involve?
SDV may be more or less thorough depending on the suspected or known quality of the data and how important data verification actually is for the specific study (more on this in the section “Drawbacks of SDV” below).
SDV primarily involves the comparison of CRF data (patient data) against source documents at trial sites, and thus primarily catches transcription errors (where data from paper records was entered incorrectly into electronic records). The main types of errors that might be found include:
- Missing data (required data fields are empty)
- Incomplete data (data that is present but not complete)
- Non-compliant data (values that are incompatible with life, unrealistic, or incorrectly formatted)
- Inaccurate data (data that doesn’t match the source document)
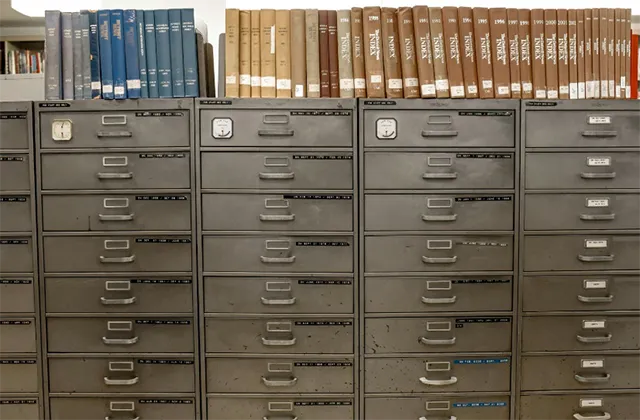
What are the benefits of SDV in clinical trials?
There are several potential benefits of performing source data verification in clinical trials, some of which we have already touched upon:
1. Data accuracy and quality
SDV can help ensure that data collected from patients in the trial is accurately reflected in the study database. By reducing errors, SDV can help to guarantee that results and conclusions drawn from a trial are sound.
2. Data consistency
SDV can improve consistency in data collected by different researchers or at different study sites, facilitating analysis of the study data.
3. Regulatory compliance and audit trails
SDV can strengthen audit trails and thus assist with regulatory compliance.
Drawbacks of SDV: Why SDV is not always necessary
Despite its potential benefits, there are also strong arguments against the necessity of full SDV, particularly amidst the new paradigm characterized by increased automation and use of coordinated electronic data systems (eClinical tools). Many such tools have built-in edit check functionalities, and beyond that, paper records are slowly being phased out in favor of direct data capture or electronic data capture (EDC).
The need for exhaustive SDV is particularly questioned in studies wherein there is already a high degree of certainty about data validity and accuracy, or where there is not an explicitly important connection between the data being verified and the trial’s research questions/conclusions or regulatory compliance. In those cases, the time and resources required to perform SDV might be better spent on other types of clinical trial monitoring.
A 2015 study published in the British Journal of Clinical Pharmacology used data from 3 phase III clinical trials to compare error dates in data that was subjected to either full or partial SDV. They found only a marginal reduction in an already low error rate, requiring verification of 370 data points to find one unspecified error. The authors argue that their results challenge the notion that exhaustive SDV is a good use of resources, particularly with the availability of other data review methods.
It’s good practice to identify risk levels concerning different types of data and to understand the specific needs of your trial in order to allocate resources efficiently. The potential benefits of complete manual SDV at sites should be carefully weighed against the time and resources required to do so. Although on-site monitoring with the aim of complete SDV was a mainstay of traditional workflows, more efficient ways to ensure data quality and demonstrate regulatory compliance have since become more common and have been proven effective.
For more in this regard, see our articles on clinical data review, as well as remote monitoring and risk-based monitoring.
Conclusions
Source data verification (SDV) is a process employed in clinical trials to check all data against source documents for accuracy and completeness. The objective of SDV is to confirm the reliability and quality of the data used in the final analysis, facilitating regulatory compliance and ensuring validity of the results. In particular, SDV is ideal for catching transcription errors, which becomes less urgent with the use of advanced software tools that are becoming commonplace in modern trial workflows.
The potential benefits of performing complete SDV for a clinical trial should be weighed against the time and resources it requires, as it may have diminishing returns. Sponsors will benefit from considering various possible solutions, including centralized or risk-based monitoring and the implementation of EDC, in order to optimize efficiency and resource utilization.